|
The DePIcT project:
Deep-Learning Data Processing of spectral PC-CT longitudinal Studies to Design and Optimize combined Immuno-Anticancer Treatments in Liver Cancer Mouse Models
Partners
This project is a collaborative study between the the imXgam team at CPPM and Flavio Maina's team at IBDM.
Context of the project
The PIXSCAN-FLI is a spectral Photon Counting micro-Computed Tomography (PC-CT) for in vivo small animal imaging based on the hybrid pixel technology developed for particle physics experiments. It includes a dedicated data processing pipeline adapted to this new physics of acquisition. The superiority of PIXSCAN-FLI with respect to other methodologies based on micro-CT, particularly its sensitivity and reliability, have been illustrated through several significant achievements among which: i) high detectability at low contrast and low X ray doses, ii) high image quality in the context of in vivo imaging, iii) fast dynamic radiographic capabilities at unprecedented acquisition rates in X-ray imaging (69 images/s and more), iv) contrast agent identification and imaging quantification. Further work concerns the implementation of deep learning state-of-the-art algorithms into the data processing and 3D reconstruction pipeline of the PIXSCAN-FLI.
Through a collaborative research between IBDM and CPPM, we recently applied the PIXSCAN-FLI methodology for in vivo imaging studies in a clinically relevant mouse model of hepatocellular carcinoma (HCC), the Alb-R26Met mice. We uncovered a remarkable heterogeneity in the dynamics for tumours at the initiation phases. Instead, the growth curve of evolving tumours exhibited a comparable exponential growth, with a constant doubling time. Furthermore, longitudinal PC-CT imaging in mice treated with a combination of MEK and BCL-XL inhibitors revealed a drastic tumour regression accompanied by a striking remodelling of macrophages in the tumour microenvironment. Collectively, these studies illustrated the capability of the PIXSCAN-FLI to perform unprecedented longitudinal studies to track spontaneous liver tumours over a timeframe period up to 3 months. Moreover, the striking remodelling of macrophages in the microenvironment of regressing tumours we reported emphasizes how imaging strategies conceived to follow distinct tissue structures - cell populations - can guide towards optimal therapeutic settings. Thus, the PIXSCAN-FLI is today a mature powerful system dedicated to small animal longitudinal imaging to detect cancer initiation and progression, and to monitor its evolution during treatment.
Main objectives of DePIcT
This project aims at exploiting the full capabilities of PIXSCAN-FLI system in a spectral mode through a novel research plan with four major objectives.
The first objective is to acquire scientific knowledge on the tumour microenvironment remodelling and to translate findings into combinatorial therapies. We will explore the effectiveness of an immuno-therapy approach designed to remodel the tumour microenvironment while targeting cancer cells with drugs. First, we will develop combinatorial PC-CT imaging acquisition and processing using different contrast agents for qualitative/quantitative evaluation and 3D reconstructions of tumours, their vascularization, and tumour microenvironment. Second, based on knowledge gathered through imaging and marker analyses, we will assess the effects of treatments combining agents acting on the immune checkpoint pathway with those targeting cancer cells. Effects will be qualitatively/quantitatively evaluated on tumour cells, tumour microenvironment, and tumour vessel network.
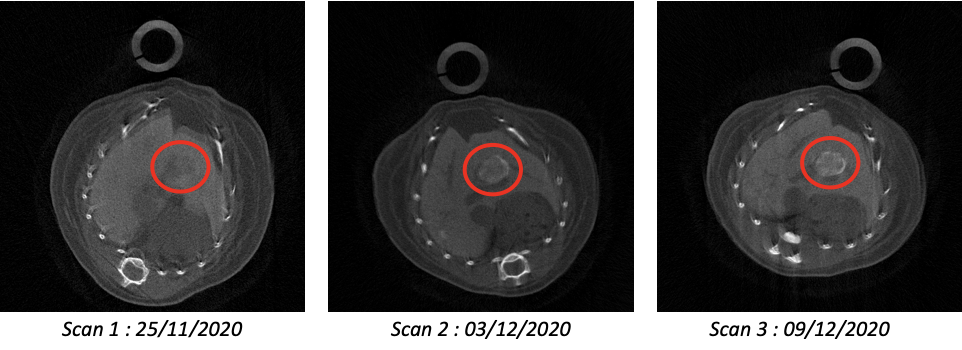 Three transverse slices of the same mouse imaged at weekly intervals.
The second objective is to extend the usefulness and relevancy of spectral CT imaging for in vivo longitudinal studies. With respect to our achievements with PIXSCAN-FLI used as a standard PC-CT, we will push one step forward the application of PIXSCAN-FLI. We will rely on the proof-of-concept showing that the use of composites pixels makes spectral in vivo imaging possible by subtracting movements. Spectral imaging will bring two valuable outputs unreachable before: 1) a precise unsupervised segmentation of the tumour, its microenvironment and vascularization; 2) quantification of macrophage areas for biological analysis.
The third objective concerns deep-learning specific developments into the PIXSCAN-FLI pipeline to optimize spectral data processing. We will focus on two points. First, we will train neural networks in order to learn all sources of noise of the system including inhomogeneity, particularly due to badly calibrated pixelsn and also to automatically perform unsupervised segmentation and classification of tumors vs. liver. Second, we will incorporate into our last published spectral data reconstruction algorithm the concept of Deep Image Prior: a neural network capable of self-configuration and reported to restore with high fidelity textures in images. This study will be a rare opportunity to assess the performances of this approach on in-vivo images.
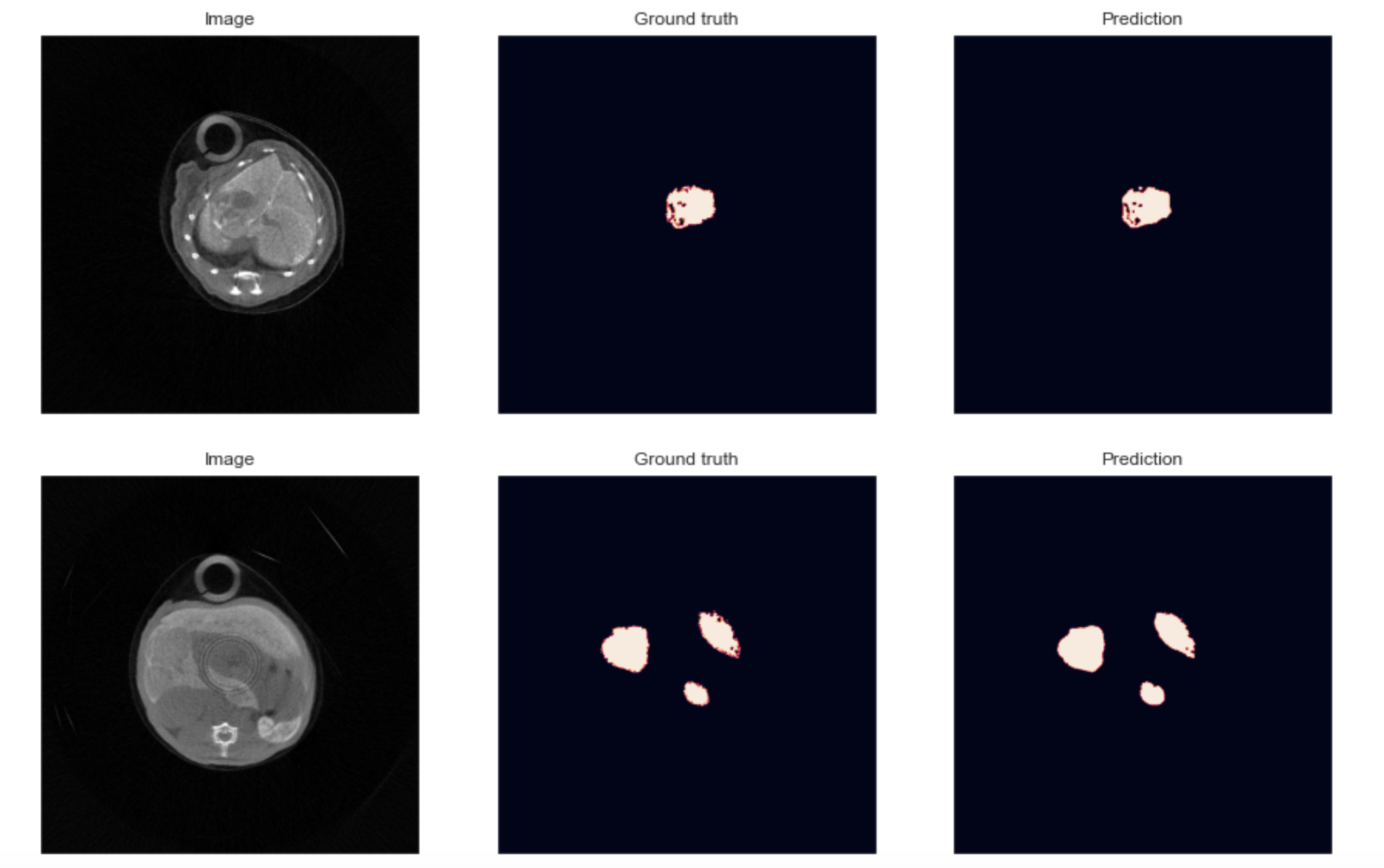 Two segmentations obtained on a Deep Learning U-net architecture on two in vivo images acquired on the PIXSCAN-FLI.
The fourth objective is to promote and share PIXSCAN-FLI application through the cancer research community using mouse models. Outcomes from our program will extend our published achievements, strengthening the usefulness of the PIXSCAN-FLI for cancer research. Members of our collaborative network and particularly the recruited PhD student will participate to national and international initiatives to transfer the knowledge on PIXSCAN-FLI applications and uniqueness. This interdisciplinary proposal combines the use of clinically relevant cancer mouse genetic models, combinatorial drug evaluation in vivo, qualitative/quantitative longitudinal in vivo imaging based on physical, mathematical, and deep learning approaches, assessment of crosstalk between cancer cells, immune cells and vessels combining imaging, histology, marker analyses. It is a highly challenging proposal, which feasibility is supported by solid competences within our network, by the availability of tools and methodologies fully established.
Publications
F. Cassol et al., Tracking Dynamics of Spontaneous Tumors in Mice Using Photon-Counting Computed Tomography, iScience 21 (2019) 68-83
|